User-defined Swarm Robot Control
Lawrence H. Kim, Daniel S. Drew, Veronika Domova, Sean Follmer

Abstract
A swarm of robots can accomplish more than the sum of its parts, and swarm systems will soon see increased use in applications ranging from tangible interfaces to search and rescue teams. However, effective human control of robot swarms has been shown to be demonstrably more diffcult than controlling a single robot, and swarm-specifc interactions methodologies are relatively underexplored. As we envision even non-expert users will have more daily in-person encounters with different numbers of robots in the future, we present a user-defned set of control interactions for tabletop swarm robots derived from an elicitation study. We investigated the effects of number of robots and proximity on the user’s interaction and found signifcant effects. For instance, participants varied between using 1-2 fngers, one hand, and both hands depending on the group size. We also provide general design guidelines such as preferred interaction modality, common strategies, and a high-agreement interaction set.
Video
Elicitation Study on In Situ Swarm Robot Control
To better understand how users prefer to interact with a swarm of robots, we conducted an elicitation study on swarm robot control. Our study results can inform what types of sensors are needed to enable fuid interaction between users and a swarm of robots. We have pre-registered our elicitation study at OSF.io (https://osf.io/r8fnc) and all raw data along with study results are freely available at https://osf.io/dkja9/ as well.
Hypotheses
H1: Number of robots will affect how users interact.
Researchers have shown that the number of robots can signifcantly alter how people perceive the robots when viewing their motion [36] or being touched by them. Researchers have also developed different ways to teleoperate or remotely control a swarm of agents such as leader-follower, selection and beacon control, and physicomimetics. Thus, we hypothesize that users will also adapt their interaction method for in situ control based on the number of robots.
H2: Proximity to the robot(s) will affect how users interact.
Literature in Human-Robot Interaction (HRI) has shown that humans perceive robots differently based on their proximity as well as prefer robots that exhibit proxemic behavior. Cauchard et al. have reported that when the robots were closer, users tended to use smaller motions. Thus, we also hypothesize that proximity to the robot(s) will change how users choose to interact with a swarm of robots.
Setup
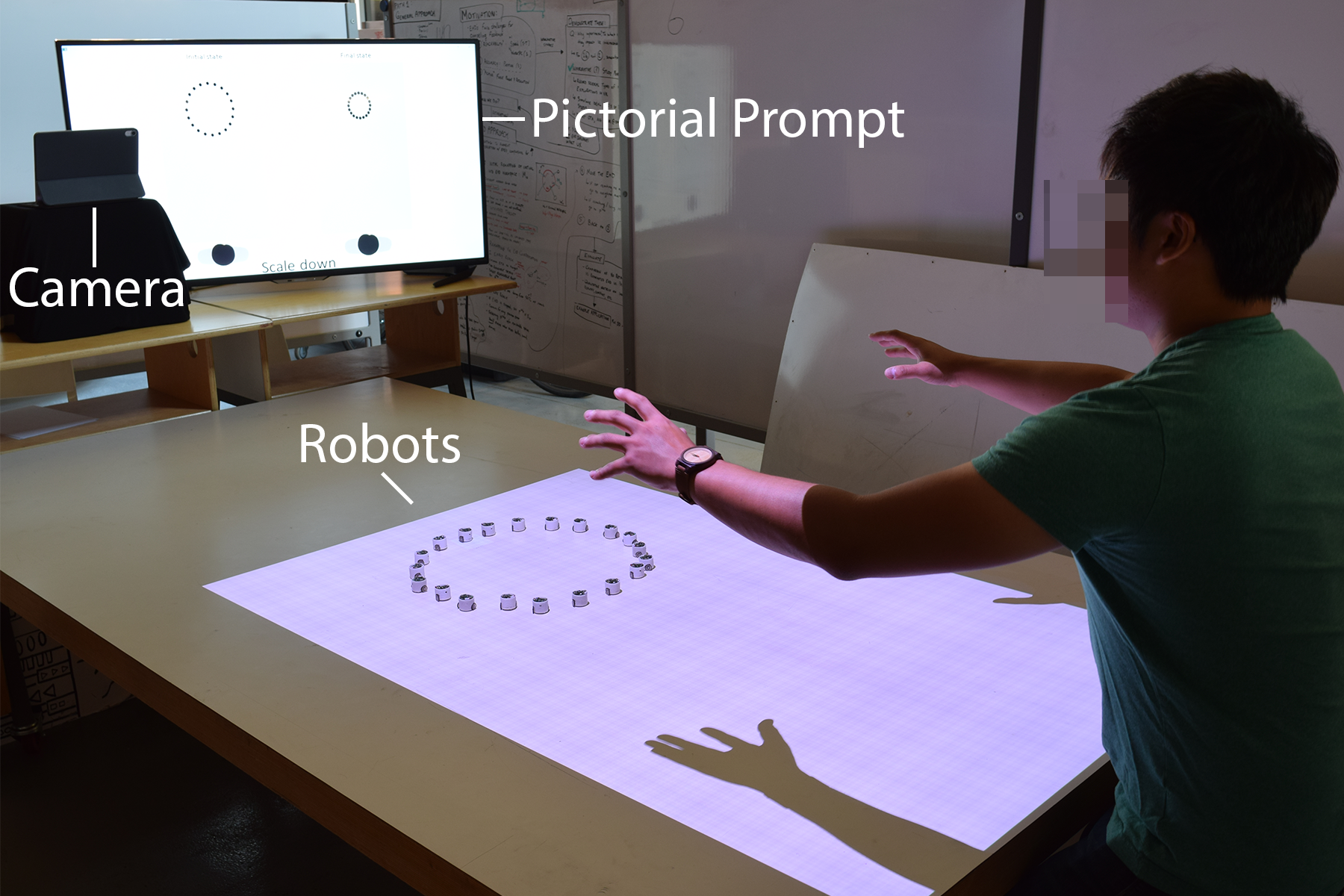
Example Prompt
Results
Interaction Modality
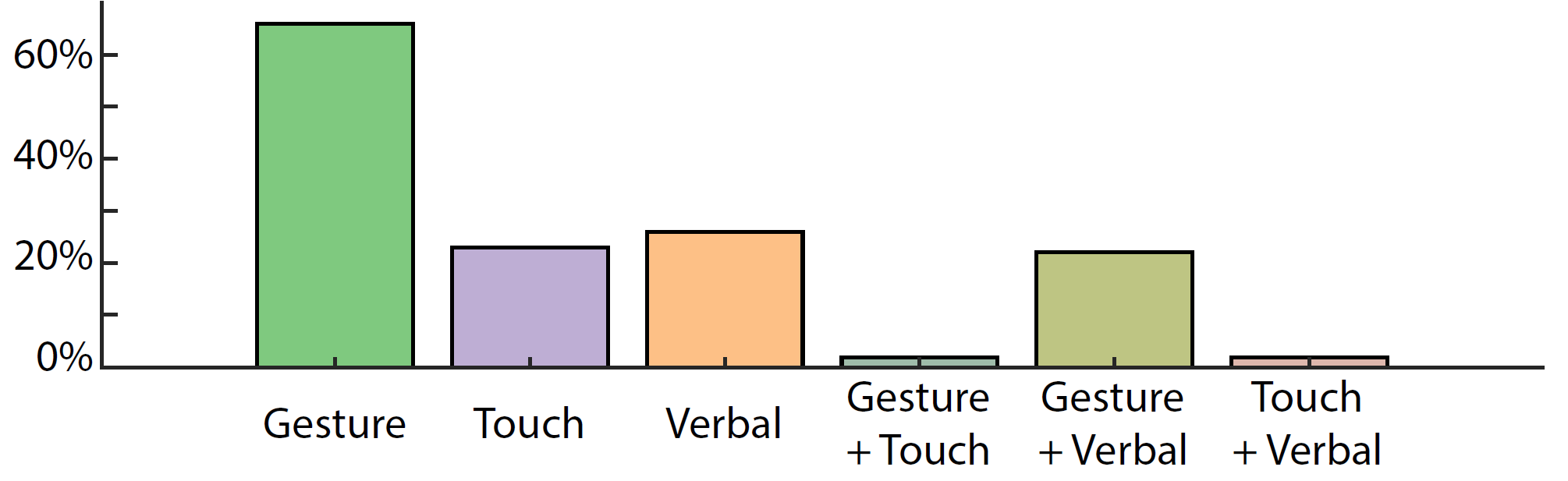
We categorized each interaction into one of the following interaction modalities: gesture, touch, verbal commands, and combinations of them. Figure 4 presents the breakdown of interaction modalities used across all conditions. For multimodal interactions, they are counted in all relevant categories. For example, interactions with both gesture and verbal commands are counted in “Gesture”, “Verbal”, and “G+V”. When looking at these overall results in the context of prior work, we see some similar trends to single robot interaction across different types of robots in terms of interaction modality - for example, our results for cm-scale wheeled robots are similar to the results found by Abtahi et al. for a single caged “safe” aerial drone [1]. Yet, our results are quite different than that of uncaged “unsafe” drones, potentially due to the non hazardous nature of our small wheeled robots. However, our results are less directly comparable to other studies which did not explore the use of touch or direct manipulation for control of many robots as the study is done in a virtual environment [17, 34]. Yet, similar to [17], we also observed that the majority of the speech commands were accompanied by a gesture.
Taxonometric Breakdown
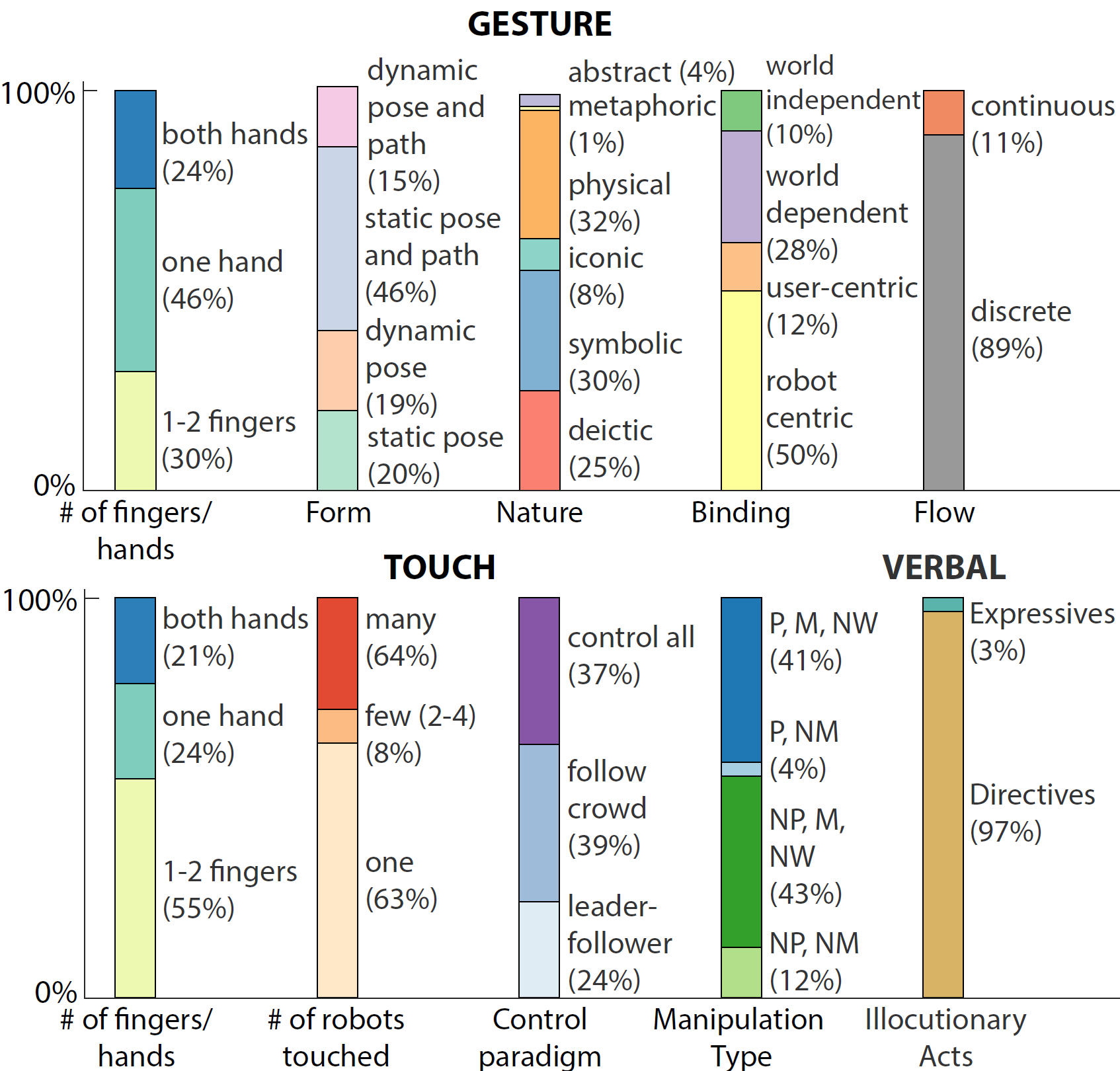
Agreement
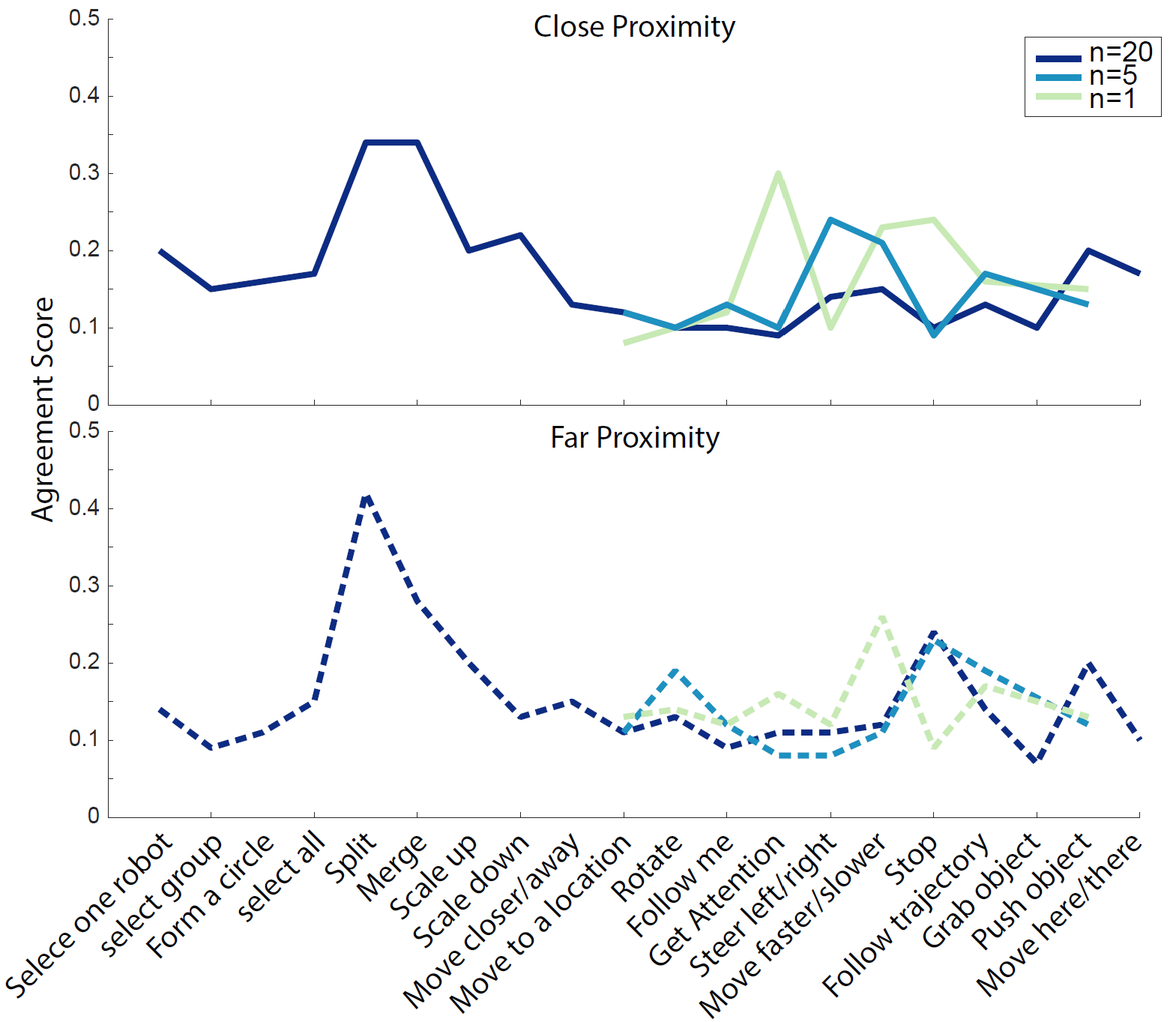
User-defined Interaction Set
The user-defned interaction set was generated by taking the most frequent interaction for each referent. If the same interaction was used for different referents thus creating confict, the interaction was assigned to the referent with the largest group.
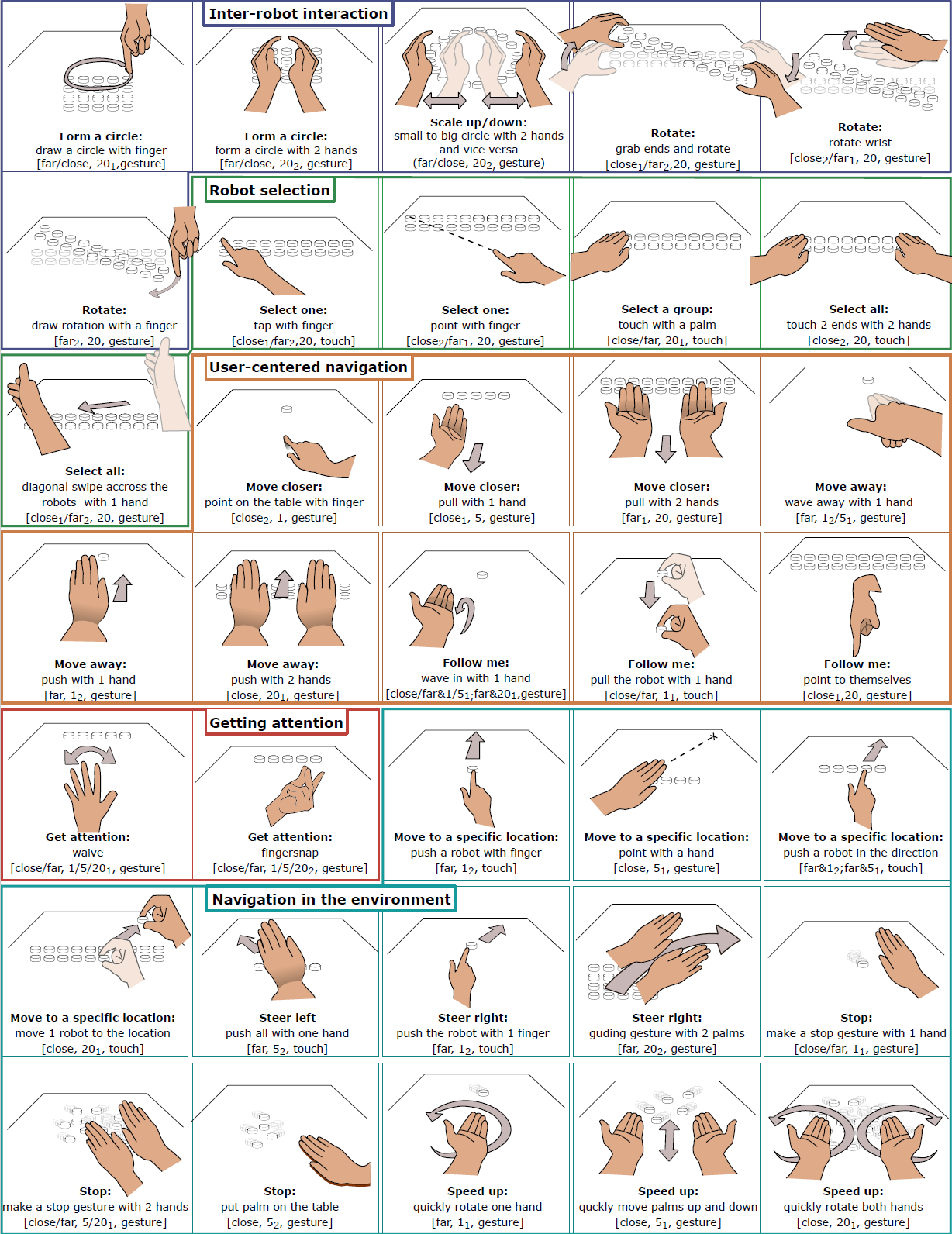
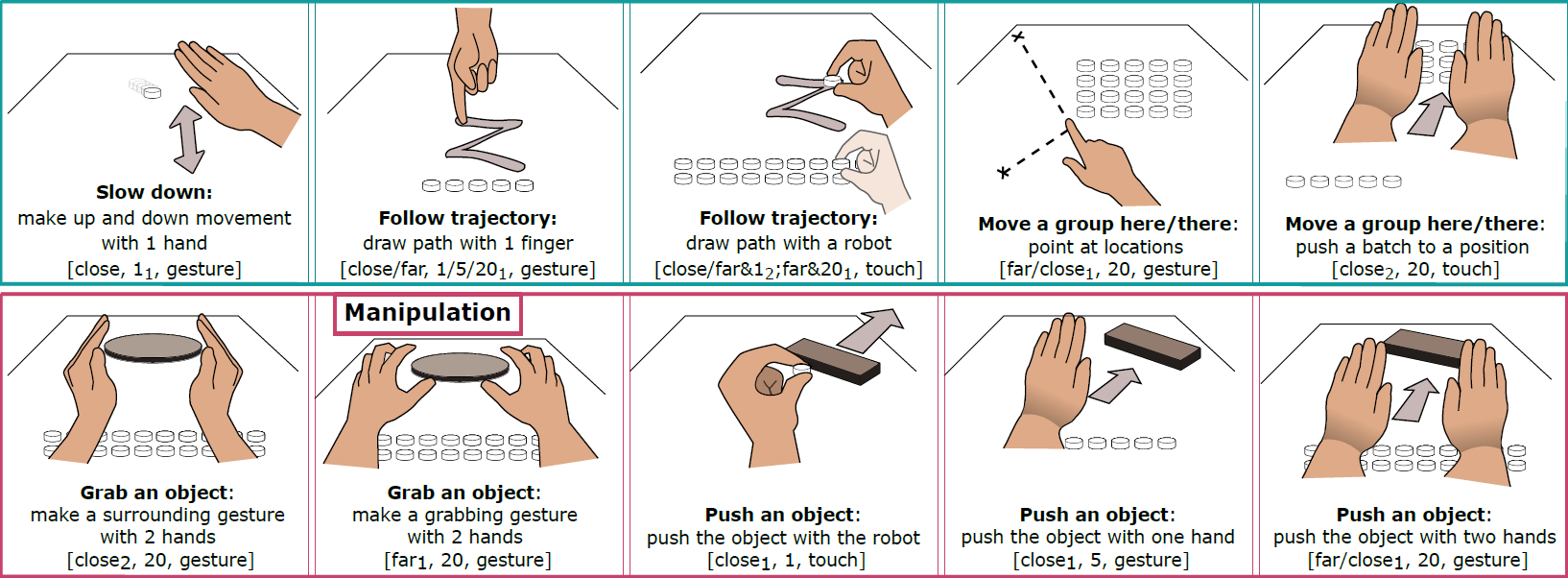
Effects of Number of Robots + Proximity to Robots
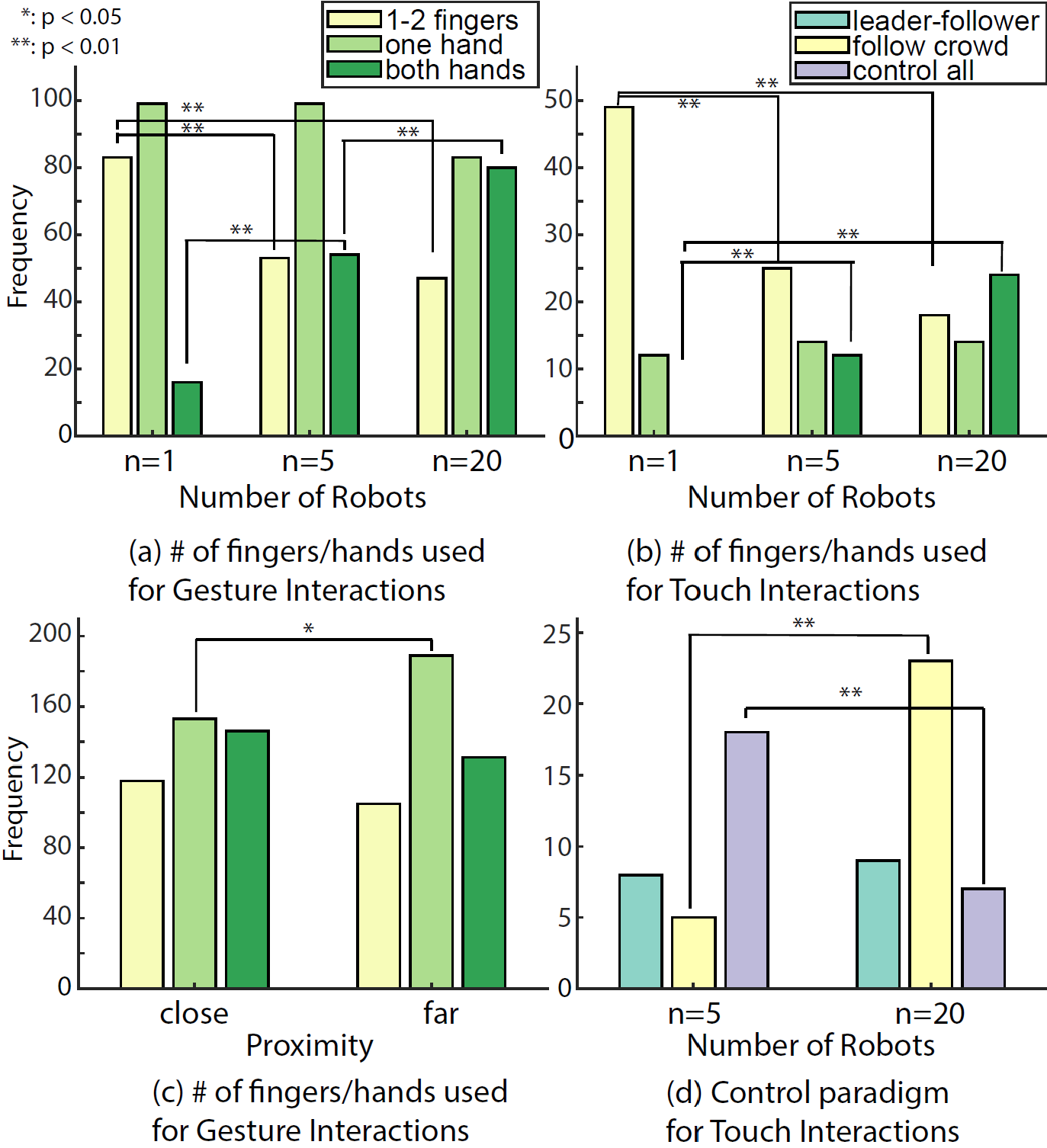
Trends within Each Referent Category
For each referent category, we compared its data with that of the remaining referents. For instance, for the robot selection category, we compared its data with that of referents not in the robot selection category.
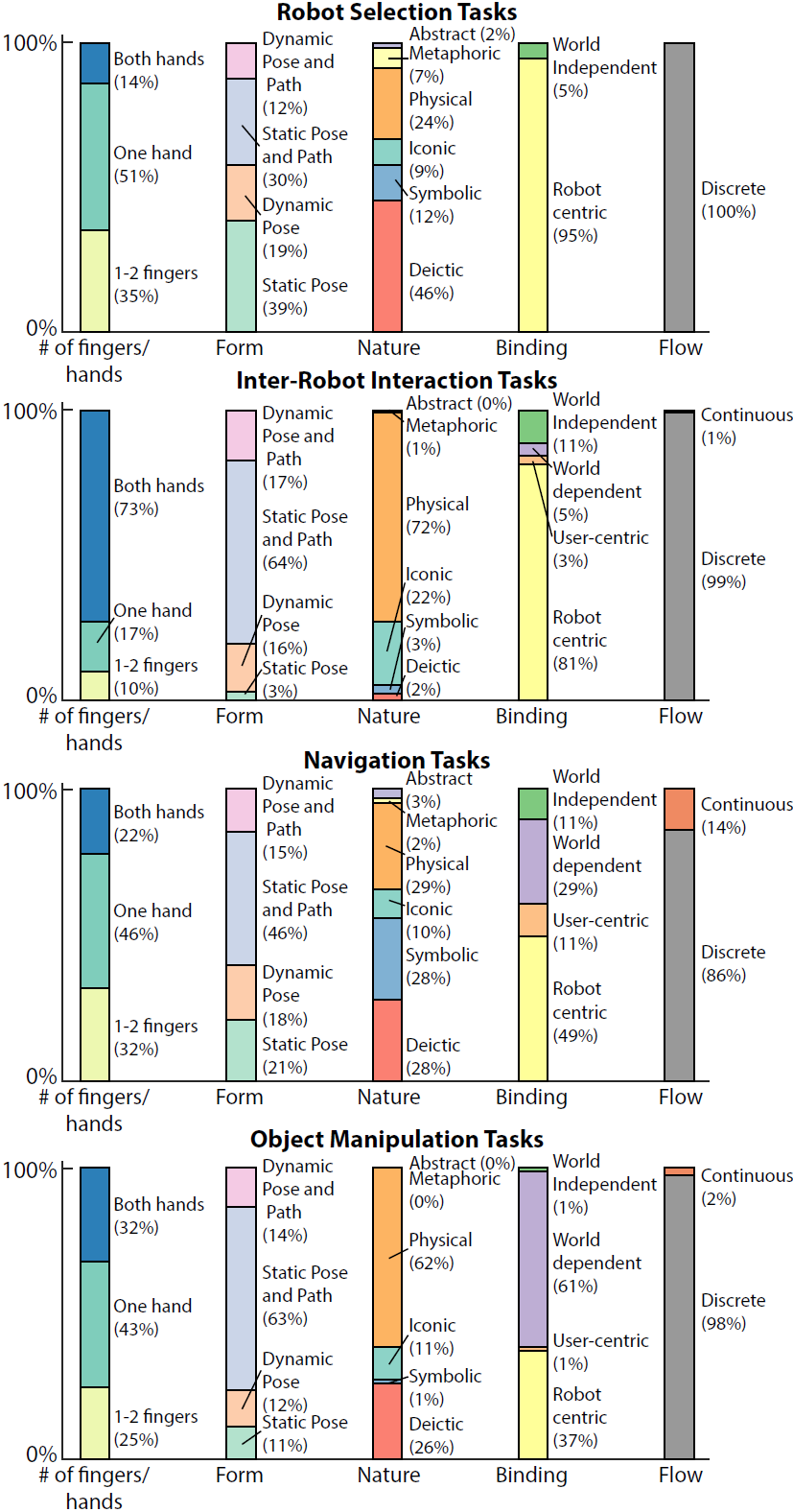
Paper
User-defined Swarm Robot Control (Best Paper Honorable Mention) [PDF]
Lawrence H. Kim, Daniel S. Drew, Veronika Domova Sean Follmer
ACM Proceedings of the 2020 CHI Conference on Human Factors in Computing Systems (CHI)